Artificial intelligence has had such a massive boom in the last year and a half, but it’s nowhere near its peak. According to a new report by Grandview Research, the global market for just the conversational AI sector of the artificial intelligence space will reach $41.39 billion by 2030. In 2022, it was just 7.61 billion.
But despite its popularity, there is still a lot of uncertainty about everything that conversational AI entails and the possibilities it’s introducing to the world. So let’s clear things up and see how this evolving tech can transform the way you, a SaaS product stakeholder, craft your product.
What is conversational AI?
Up until just recently, the popular discourse simply referred to artificial intelligence broadly. But as the tech evolves, the need to refer to the subsections of AI grows. The two most popular branches under the umbrella of AI are generative AI and conversational AI. Let’s dig deeper into the second one.
The goal of conversational AI is to mimic human interactions so that you can scale human-like experiences without needing tons of people resources. This branch of AI refers to the technologies that enable computers to simulate these real conversations with humans by creating algorithms that can understand, process, and respond to human language as naturally as possible. Machine learning uses tons of training data to inform the best responses and can even learn from interactions to improve over time with data labeling playing a crucial role in ensuring the accuracy and relevance of this training data.
These conversations can either be in text or spoken form.
Are chatbots and conversational AI the same thing?
Many people may incorrectly use chatbots and conversational AI interchangeably. And this is understandable. On its face, the end user experience at a high level may seem the same: interacting through dialogue. However, the depth of the conversations as well as the tech underlying them can be very different.
In the OG chatbots, there was no AI at all. They were simply rule-based bots that followed a set of scripted responses to pre-defined queries.
Now, some (but not all) chatbots do use conversational AI to create a more natural-feeling, dynamic chat experience that can also dig into more unexpected responses. But the thing to take away here is that conversational AI is what powers these chatbots. Think of it like the chatbot being the vehicle and conversational AI being the engine.
History of conversational AI
Time for a fun history lesson on how far conversational AI has come from the early days of computer science.
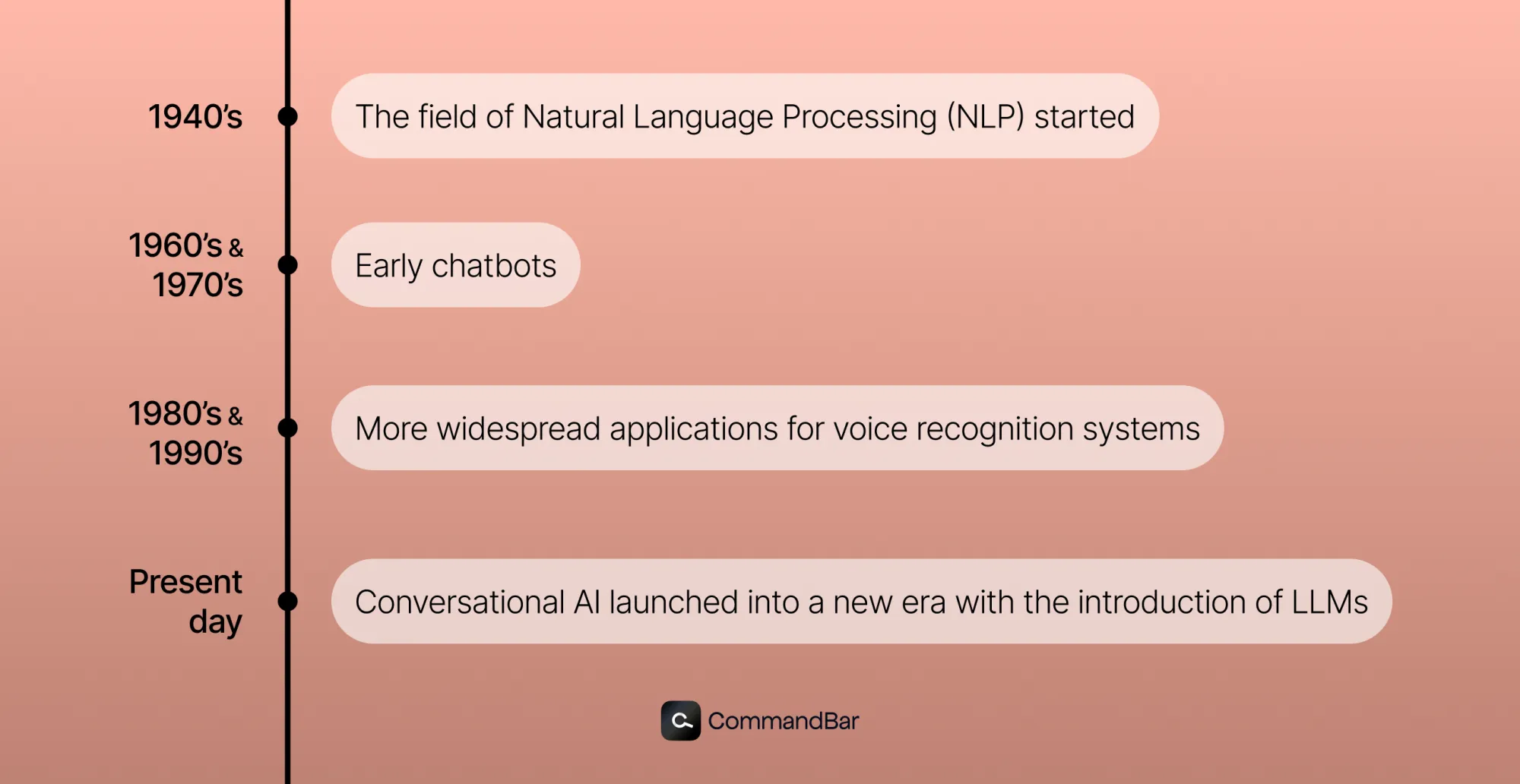
After World War II, there was a big demand for technology that can automatically translate between different languages to make communicating globally easier. And so began the field of Natural Language Processing, or NLP as you may have heard it referred to as. This field of study is all about getting computers to understand and respond to human language.
Early chatbots in the 1960’s and 70’s
In the early 70’s, the very first chatbot named ELIZA was launched. “She” used pattern matching and substitution methodology to simulate conversation based on a script called DOCTOR, because it mimicked a psychotherapist’s conversational style.
However, Eliza mainly worked by rephrasing much of what people on the other end said to her back to them. This gave an illusion of understanding, but it lacked a true understanding of the language of context. This signaled future concerns about biases in AI, which we’ll get more into later, since even these early models merely reflected and synthesized, rather than drawing unique conclusions.
And then along came PARRY, nicknamed “ELIZA with attitude.” But the outcomes were still not revolutionary. Want some proof? Just take a look at a snippet of the comical conversation between the two bots that sounds like an exchange between a kooky patient and the world’s worst therapist.
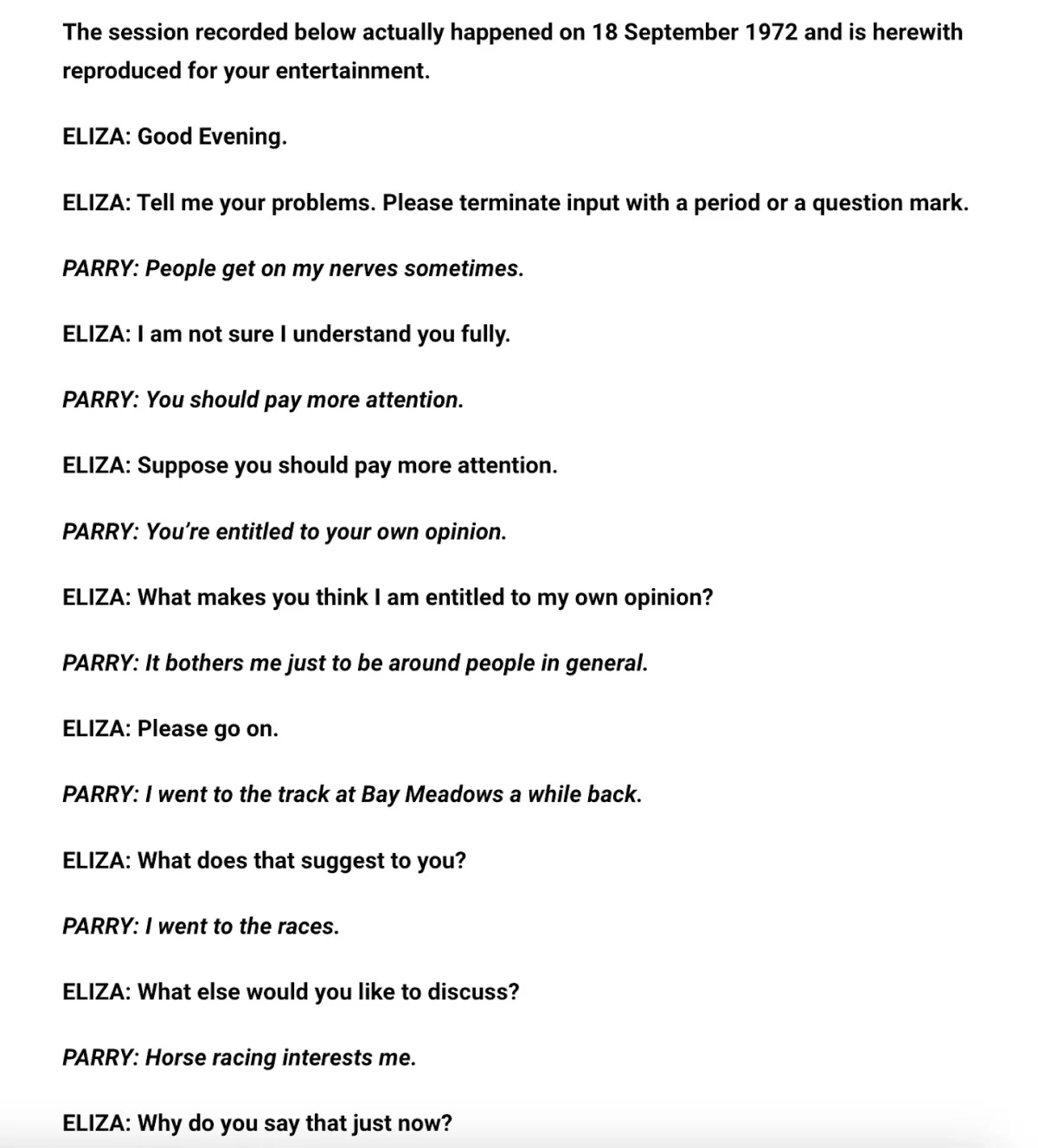
Slightly more advanced tech in the 1980’s and 1990’s
Sophisticated systems began to come together thanks to the development in computational power and algorithms at the end of the 20th century. This is when conversational AI began to move out of just the theoretical and academic contexts and into more widespread practical uses.
Interactive voice response (IVR) systems began to pop up in the 80’s. These systems helped businesses handle large volumes of phone calls, especially in industries like banks and airlines. These used basic speech recognition software to shift some of the burden away from phone agents.
There were also early attempts at voice commands in operating systems. In the early to mid-90’s both Microsoft Windows and Apple Macintosh integrated capabilities to recognize voice commands for basic tasks like opening files or programs. Although Apple’s PlainTalk is very different from Siri today, you could consider it a rudimentary precursor for what’s to come.
Dictation also experienced a lot of growth during the early 90’s. Dragon Dictate was one of the first commercially successful speech recognition programs that let people talk to type text on their computer. This was a big win for productivity in general, but especially for disabled users.
And finally, towards the end of these decades, things started to get a little more human. Julie was Amtrak’s automated voice agent that could direct calls and provide automated interactions. But the most notable thing about her is how “human” she felt. For the first time, people were using words like “spunky,” “reassuring,” “perky,” and “courteous” to describe technology. Amtrak’s ability to set the standard for humanity in conversational AI has been pinned down as one of the biggest reasons for the success of the company.
Everything changed once we began to integrate context and memory through LLMs
The real turning point in conversational AI came with the introduction of systems capable of maintaining context over the course of a conversation, allowing for more meaningful and sustained interactions.
These systems began to use more complex models to manage dialogue states and recall past interactions, setting the stage for the truly intelligent systems that we have today and will continue to evolve into in the future.
And much of the progress we’ve made is thanks to the development of LLM’s in just the last decade, so we’re in a really exciting time.
So what is an LLM?
An LLM, or Large Language Model, is an AI model trained on vast amounts of text to understand and generate human-like language. It uses this training to perform tasks like answering questions, translating languages, and more.
There are several popular LLMs today, like OpenAI’s GPT (Generative Pre-Trained Transformer) and Google’s BERT (Bidirectional Encoder Representations from Transformers). Although the names may not be the most exciting you’ve ever heard, they have completely accelerated the growth of conversational AI as a discipline.
The creation of LLMs actually has its roots in the study of the nervous system. The artificial neural networks (ANNs)that back up these models mimic the structure and function of the human brain. So that means that we’re creating genuinely INTELLIGENT machines.
This brain-like function of LLMs helps to integrate the contextual understanding and memory that is needed for these machines to truly understand and interact in a human-like way. It’s now increasingly possible for conversational AI machines to grasp the finer nuances of language, interpret complex questions, and provide more contextually relevant and coherent responses.
So you’ve made it to the end of your 101-level crash course of conversational AI, but you may still be unclear about the extent to which this can be leveraged in SaaS. Spoiler: it’s transformative.
The biggest opportunities for conversational AI in SaaS
In the next decade and beyond, there may be countless other opportunities for conversational AI to bring SaaS products to the next level (or to resolve operational inefficiencies), but as of now, there are 3 clear trends emerging.
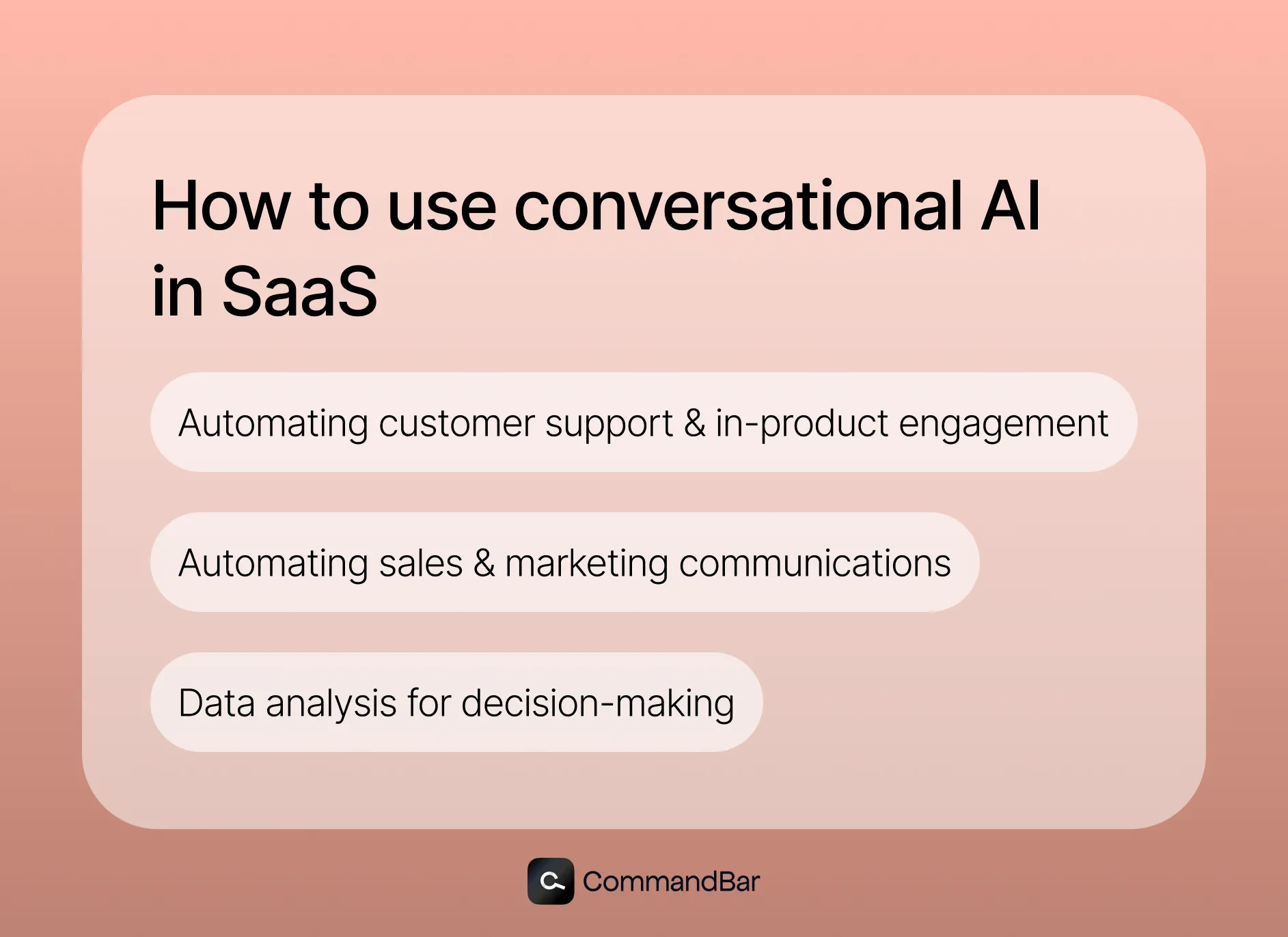
Customer support and customer experience automation
Conversational AI can help to shift some of the time-consuming tasks away from your human support team and to technology. This helps to automate some of the simpler tasks and frees your team up to have more time to spend on complex tasks or relationship-building.
This is especially helpful if you have a high volume of support tickets, but it can also be transformative in streamlining onboarding and helping your users master your platform.
AI-powered Virtual support agents like Command AI’s Copilot goes bound beyond simplistic chatbots. It allows users to get the best of both worlds when it comes to timely self-service and reliable support. Users are able to ask the virtual agent any question, in their own language, and get easy-to-understand answers back immediately.
This means that users won’t have to be frustrated with chatbots, whether AI-based or rule-based, that just aren’t understanding. But they also won’t have to wait for a human agent to be available. Instead, they get 24/7 access to a virtual product assistant that's ready to help them with any question, concern, or curiosity.
Conversational AI can also be used to boost in-app productivity. Notion’s new AI is the perfect example of this. Since many people use Notion as their company wiki, that means there’s loads of information stored in there. But users often struggle to access the right information when they need it, leading to time wasted searching for files or duplicate files getting uploaded.
With Notion AI, people can ask questions about the data stored in their workspace. For example, maybe someone wants to know what the HEX code for their logo color is, their company’s mission statement, or whether a certain item can be filed for reimbursement. Instead of having to ask team members or search through several files, they can just ask the question to the bot, which intelligently probes the existing information to get an answer and even provides insight into the sources it used.
Sales and marketing automation
SaaS support teams aren’t the only ones who may struggle to keep up with the volume of work needed to be done to help the company grow. Sales and marketing teams can also benefit greatly from conversational AI tech that helps them scale their communication.
These tools allow “you” to chat with leads in real-time. You’ve probably interacted with one of these on-page chats like this before, but whether it was built on a solid LLM may have shaped whether that experience was positive or negative.
The most intelligent conversational AI tools can automatically and empathetically engage with browsers on your website. The personalization of these chats can be done on the page level (i.e. you start the conversation different for someone on the pricing page vs. a certain features page) and/or on the individual user level (i.e. the messages that resonate with a brand new users will be different from those that spur a user who has repeatedly looked at valuable pages to action).
Conversational AI tools can help to engage new leads immediately and continuously nurture existing leads. You can also create a good relationship between these tools (your virtual sales team) and your human sales team by baking in triggers that indicate a lead is hot and should be shifted over to a salesperson.
Data collection and analysis
You can also use all of the conversational data that you’re collected across the different AI conversational AI tools you implement to fuel decision-making.
Conversational analytics is a growing field that helps you analyze all of this interaction data to identify trends, predict customer needs or behavior, and refine your customer profiles, for example, so that you can tailor your products more effectively. Overall, it helps with better decision-making.
Say, for example, that complaints or support tickets are growing for a certain product or feature. Using your conversational data, you can get alerted that an issue may be arising as well as dig into additional context that your users are providing surrounding that issue. This helps you to be proactive about resolving issues before they reach an inflection point.
The future of conversational AI
This era of conversational AI is relatively new, especially in SaaS products. So although we’ve already come so far in the last decade, the growth is likely to continue on an exponential path.
Here are some things to keep an eye out for as we move forward into the years or even months ahead.
Innovations on the horizon
Emotional intelligence is an increasingly bigger priority for conversational AI models and can help to take these tools to the next level now that we’ve achieved contextual understanding and memory. Emerging models are beginning to interpret emotional cues in both text and voice, which can lead to more empathetic and genuine-feeling interactions.
Some cloud contact center platforms have already adopted this technology, leveraging sentiment analysis within their conversational AI systems. This allows them to better understand customers' moods, respond more effectively to inquiries, and quickly address any misunderstandings, ultimately enhancing the overall customer experience.
Most impressively, some models are now able to pick up on more nuanced emotions like sarcasm, as well as express it back. This helps these tools feel more human by picking up on some of the trickier subtleties of conversation.
Multilingual support is another major priority, and we’re making some headway. More advanced systems are being developed that can seamlessly switch between languages to serve an increasingly global customer base.
Being an early adopter of these tools can be a major opportunity for SaaS companies to differentiate themselves. 75% of consumers from non-English speaking countries prefer to make a purchase in their own language. Multilingual conversational AI can help companies meet this need without the necessity for hiring multilingual team members.
Challenges and considerations
There are also some major challenges going forward as the technology becomes more advanced.
Ethical considerations are at the forefront of many conversations about AI. There are concerns about algorithms amplifying existing biases in anything from hiring processes to content creation. There are emerging schools of thought to combat these biases and prevent the deepening of discrimination and stereotype perpetuation along racial, gender, or disability lines.
Another ethical concern is misinformation. Although this is arguably more of an issue in generative AI, where many thought leaders are at risk of blindly believing AI hallucinations in the content they’re creating, this could make its way into conversational AI in SaaS platforms as well.
Privacy concerns are another major consideration for AI companies as well as companies that are using AI. Since there is so much information being collected from users during these artificial conversations, it opens you up to risk of personal information and data being stolen in data breaches or cyber-attacks.
Companies must also consider whether their data is being used to train future conversations, potentially revealing intellectual property.
Conversational has come a long way, and it has a long way to go
Compare the first AI-powered chatbot to the almost human-like conversations we’re able to have with even the most simplistic conversational AI tools we have today. The progress is astounding. And it signals that we probably can’t even fathom the potential that these tools will have years down the road.
Your responsibility as a SaaS innovator, whether you’re a founder, product manager, or the person in charge of support operations, is to continuously think critically about ways you can leverage conversational AI to not only help your team work more efficiently but perhaps more importantly, to give your users the ability to interact more intuitively and meaningfully with your product.